Data-Driven Workforce Management: Optimizing Staffing Models in Multinational Contact Centers
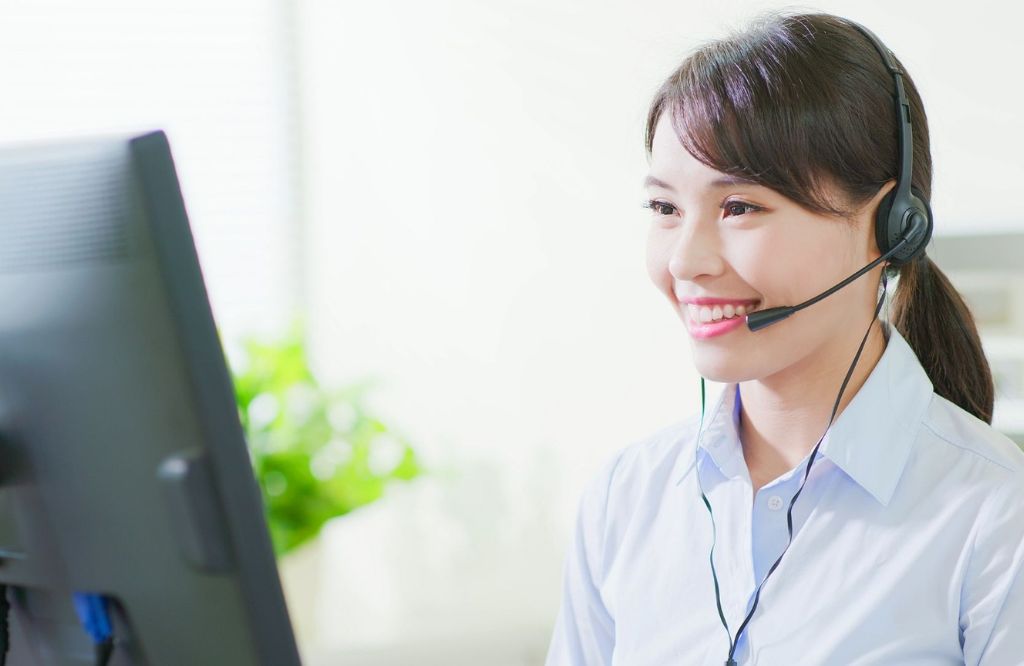
Contact centers have evolved from simple customer service channels into sophisticated customer experience hubs that directly impact brand perception, customer loyalty, and ultimately, revenue. For organizations leveraging BPO services across multiple countries, effective workforce management has emerged as a critical differentiator between operational excellence and costly inefficiency.
The traditional approach to service provider staffing—relying primarily on historical patterns and managerial intuition—is increasingly inadequate in the face of complex, multinational operations. Modern contact centers, particularly those with nearshore operations in locations like Colombia, must navigate fluctuating call volumes, evolving customer expectations, and diverse workforce dynamics across multiple time zones and cultural contexts.
Data-driven workforce management offers a powerful alternative, leveraging advanced analytics and machine learning to optimize staffing models with unprecedented precision. By transforming vast amounts of operational data into actionable insights, organizations can align their human resources exactly where and when they’re needed, dramatically improving both operational efficiency and customer experience.
This article explores the evolution, methodology, and implementation of data-driven workforce management in multinational outsourcing companies, with particular focus on optimizing operations across diverse geographic locations. Through a systematic approach to workforce data analysis and modeling, organizations can achieve the elusive balance of operational excellence and cost efficiency that defines world-class contact center operations.
The Evolution of Contact Center Workforce Management
To understand the transformative potential of data-driven approaches, it’s helpful to examine how contact center workforce management has evolved over time:
First Generation: Basic Scheduling
The earliest approach to BPO staffing focused almost exclusively on schedule adherence and occupancy rates. Managers created fixed schedules based on historical call volumes, with minimal flexibility to accommodate real-time fluctuations. Performance was measured primarily through efficiency metrics like average handle time and calls per hour, with limited consideration of quality or customer experience.
This approach worked reasonably well in simple, single-location operations with predictable call volumes. However, it proved woefully inadequate for multinational operations where complexity increased exponentially with each additional location and service line.
Second Generation: Erlang-Based Forecasting
The introduction of Erlang C calculations in the 1980s and 1990s represented a significant advancement, enabling more sophisticated forecasting based on call arrival patterns, handle times, and service level objectives. This mathematical approach allowed managers to predict staffing needs with greater accuracy, reducing both overstaffing and understaffing scenarios.
While Erlang models improved forecasting accuracy, they still relied on relatively simplistic assumptions about call arrival patterns and agent productivity. They also failed to account for the increasing complexity of multichannel interactions and the unique dynamics of geographically distributed operations.
Third Generation: Integrated Workforce Management Systems
The early 2000s saw the emergence of integrated workforce management (WFM) systems that combined forecasting, scheduling, and performance management in comprehensive software platforms. These systems incorporated more sophisticated algorithms and began to account for factors like agent skills, channel preferences, and seasonal variations.
This generation represented a significant improvement, particularly in handling the complexity of multichannel operations. However, most systems still operated primarily on historical data and struggled to adapt quickly to emerging patterns or unexpected events.
Fourth Generation: Data-Driven Workforce Optimization
Today’s leading organizations have moved beyond traditional WFM to embrace truly data-driven workforce optimization. This approach leverages advanced analytics, machine learning, and real-time data processing to create dynamic staffing models that continuously adapt to changing conditions. Rather than simply reacting to historical patterns, these systems identify emerging trends, predict future needs, and proactively optimize resource allocation across complex multinational operations.
The fourth generation represents a fundamental shift from workforce management as an administrative function to a strategic capability that directly impacts business outcomes. Organizations that successfully implement data-driven approaches gain significant competitive advantages in both operational efficiency and customer experience quality.
The Core Components of Data-Driven Workforce Management
Effective data-driven workforce management in multinational contact centers encompasses several interconnected components:
Multidimensional Forecasting
Traditional forecasting focused primarily on call volumes by time interval. Modern data-driven approaches incorporate multiple dimensions to create more accurate and nuanced predictions:
Channel-Specific Modeling: Separate forecasting models for voice, chat, email, social media, and other channels, each accounting for the unique handling characteristics and arrival patterns of that channel.
Interaction Complexity Analysis: Prediction of not just volume but complexity distribution, enabling more precise staffing based on the expected difficulty and duration of interactions.
Seasonal and Cyclical Pattern Identification: Sophisticated pattern recognition that identifies and accounts for multiple overlapping cycles, from daily and weekly patterns to monthly, seasonal, and annual trends.
External Factor Correlation: Integration of external variables like marketing campaigns, product launches, system outages, or even weather events that might impact contact volumes and types.
Cross-Channel Impact Analysis: Modeling of how changes in one channel affect others, such as how website issues might drive increased call volumes or how chat availability influences email submissions.
For multinational operations, these forecasting dimensions must be further segmented by location, language, and time zone to create truly actionable predictions. A contact center operation in Colombia, for instance, might require different forecasting models than one in the Philippines due to distinct customer bases, language requirements, and operational patterns.
Dynamic Skill-Based Routing
Beyond simply predicting volume, data-driven workforce management optimizes how interactions are distributed across available agents:
Comprehensive Skill Mapping: Detailed tracking of agent capabilities across multiple dimensions, including technical knowledge, language proficiency, communication style, problem-solving ability, and customer handling skills.
Performance-Based Routing: Allocation of interactions based not just on availability but on demonstrated performance with similar interaction types, continuously updated based on quality scores and outcome metrics.
Real-Time Adaptation: Dynamic adjustment of routing rules based on current conditions, such as unexpected volume spikes, agent availability changes, or emerging issue patterns.
Predictive Matching: Use of machine learning algorithms to identify optimal agent-customer pairings based on interaction characteristics, customer history, and agent performance patterns.
This sophisticated routing capability is particularly valuable in multinational operations where agent skills may vary significantly across locations. For example, a nearshore operation in Colombia might have particular strengths in Spanish-language service and cultural affinity with North American customers, making it ideal for specific interaction types.
Precision Scheduling
Data-driven scheduling moves beyond basic shift patterns to create optimized staffing models:
Micro-Interval Scheduling: Staffing calculations at 15-minute or even 5-minute intervals rather than hourly blocks, enabling more precise alignment with predicted demand patterns.
Flexible Shift Construction: Dynamic creation of shift patterns optimized for both operational needs and agent preferences, moving beyond rigid templates to personalized schedules.
Skills-Based Scheduling: Allocation of shifts based not just on volume requirements but on the specific skill needs predicted for each time period, ensuring the right capabilities are available when needed.
Preference-Driven Assignments: Incorporation of agent preferences and performance patterns into scheduling decisions, recognizing that agents perform better during their optimal working hours and when handling their preferred interaction types.
For business services operations spanning multiple countries, precision scheduling must also account for time zone differences, local labor regulations, cultural holidays, and regional work patterns. A sophisticated data-driven approach can navigate these complexities to create globally optimized schedules that respect local constraints.
Real-Time Management
Perhaps the most transformative aspect of data-driven workforce management is the shift from static planning to dynamic, real-time optimization:
Continuous Forecast Adjustment: Real-time updating of volume and complexity predictions based on emerging patterns, with corresponding adjustments to staffing recommendations.
Intraday Reoptimization: Automated reallocation of resources throughout the day in response to changing conditions, such as unexpected volume spikes, higher-than-predicted handle times, or agent absences.
Exception-Based Management: Intelligent alerting that focuses supervisor attention on significant deviations from plan rather than routine variations, enabling more efficient performance management.
Predictive Adherence Management: Identification of potential adherence issues before they impact service levels, with automated interventions to maintain optimal staffing.
This real-time capability is especially critical in multinational operations where supervisors may be managing teams across multiple locations. By automating routine adjustments and highlighting only significant issues, data-driven systems enable more effective management of geographically distributed teams.
Implementing Data-Driven Workforce Management: A Methodical Approach
Transitioning to truly data-driven workforce management requires a systematic approach, particularly in complex multinational environments:
Phase 1: Data Foundation
The journey begins with establishing a robust data foundation:
Data Inventory and Quality Assessment: Comprehensive evaluation of available data sources, including ACD records, quality monitoring scores, customer feedback, and operational metrics. This assessment should identify gaps, quality issues, and integration challenges that need to be addressed.
Unified Data Architecture: Development of a centralized data repository that integrates information from multiple systems and locations, creating a single source of truth for workforce analytics. This architecture must accommodate the diverse systems often found in multinational operations while ensuring consistent data definitions and relationships.
Historical Data Enrichment: Enhancement of historical data with contextual information such as marketing activities, system changes, or external events that might explain anomalous patterns. This enrichment provides critical context for model development and validation.
Data Governance Framework: Establishment of clear processes for data collection, validation, storage, and access, ensuring that workforce analytics are based on reliable, consistent information. This framework should include specific provisions for handling data across international boundaries in compliance with relevant privacy regulations.
For contact center operations spanning multiple countries like Colombia and other nearshore locations, this data foundation must accommodate differences in systems, metrics, and operational practices while creating a unified analytical environment.
Phase 2: Analytical Model Development
With a solid data foundation in place, organizations can develop the analytical models that drive workforce optimization:
Volume and Pattern Analysis: Deep examination of historical interaction patterns across channels, time periods, and locations to identify underlying drivers and relationships. This analysis should go beyond simple averages to understand variability, correlations, and anomalies.
Predictive Model Construction: Development of forecasting models that incorporate multiple variables and techniques, from traditional time series analysis to machine learning approaches. These models should be tailored to the specific characteristics of each channel, location, and customer segment.
Agent Performance Modeling: Creation of comprehensive agent performance profiles that identify individual strengths, development areas, and optimal working conditions. These profiles should incorporate both efficiency metrics and quality indicators to provide a balanced view of performance.
Scenario Simulation Capability: Implementation of simulation tools that can model the impact of different staffing configurations, routing strategies, and scheduling approaches. These simulations enable data-driven decision-making about operational changes before implementation.
The model development process should be iterative, with continuous validation against actual results and regular refinement based on emerging patterns. For multinational operations, models must account for location-specific factors while enabling enterprise-wide optimization.
Phase 3: Operational Integration
Analytical models deliver value only when they’re effectively integrated into operational processes:
Workflow Redesign: Reconfiguration of planning, scheduling, and management workflows to incorporate data-driven insights and recommendations. This redesign should streamline processes while ensuring appropriate human oversight of system recommendations.
User Interface Development: Creation of intuitive interfaces that present complex analytical insights in actionable formats for different user roles, from executives to supervisors to agents. These interfaces should highlight the most relevant information for each role while providing drill-down capabilities for deeper analysis.
Exception Management Framework: Establishment of clear thresholds, alerts, and escalation processes for managing deviations from forecast or performance expectations. This framework should distinguish between routine variations and significant issues requiring intervention.
Change Management Program: Comprehensive approach to helping stakeholders understand, accept, and effectively use data-driven tools and insights. This program should address both technical skills and potential resistance to new ways of working.
For contact centers with operations in Colombia and other international locations, operational integration must respect local management practices while ensuring consistent application of data-driven principles across the enterprise.
Phase 4: Continuous Optimization
Data-driven workforce management is not a one-time implementation but an ongoing capability:
Performance Monitoring System: Regular evaluation of forecast accuracy, scheduling efficiency, and operational outcomes against established targets. This monitoring should identify both successes and opportunities for improvement.
Model Refinement Process: Systematic approach to updating analytical models based on new data, emerging patterns, and changing business requirements. This process should include both automated adjustments and periodic comprehensive reviews.
Innovation Pipeline: Structured approach to testing and implementing new analytical techniques, data sources, or operational approaches. This pipeline ensures that the organization continues to advance its capabilities rather than stagnating after initial implementation.
Cross-Location Learning: Mechanisms for identifying and sharing best practices across different contact center locations, creating a virtuous cycle of improvement. This learning should flow in multiple directions, recognizing that valuable innovations can emerge from any location.
By establishing these continuous optimization mechanisms, organizations can ensure that their data-driven workforce management capabilities remain effective as business needs, customer expectations, and analytical possibilities evolve.
Multinational Financial Services Provider
A leading financial services company with outsourcing provider operations across multiple countries, including a significant nearshore presence in Colombia, provides an instructive example of data-driven workforce management implementation.
The organization faced several challenges common to multinational contact centers:
- Inconsistent performance across locations, with service levels varying significantly by country
- Difficulty aligning staffing with demand across multiple time zones and languages
- Inefficient allocation of interactions, with some agents overwhelmed while others were underutilized
- Limited visibility into the drivers of performance differences between locations
After assessing these challenges, the company implemented a comprehensive data-driven workforce management approach:
Unified Data Platform: They created a centralized analytics environment that integrated data from all locations, channels, and systems, providing a single source of truth for workforce decisions. This platform standardized metrics and definitions across countries while accommodating necessary local variations.
Advanced Forecasting Models: The company developed location-specific forecasting models that incorporated not just historical patterns but external factors like marketing campaigns, system changes, and even local events in each country. These models achieved 95%+ accuracy at the 15-minute interval level, a significant improvement over their previous 80% accuracy.
Skills-Based Optimization: They implemented sophisticated skills mapping and performance tracking that identified the unique strengths of each location. This analysis revealed that their contact center in Colombia excelled at complex problem-solving and relationship building, while other locations had different specializations.
Dynamic Routing Engine: Based on this skills mapping, they deployed an intelligent routing system that directed interactions to the optimal location and agent based on type, complexity, language, and customer value. This engine continuously learned and adapted based on performance outcomes.
Flexible Scheduling Framework: The company moved from rigid shift patterns to a more flexible approach that optimized staffing across all locations while respecting local labor regulations and work preferences. This framework included options for remote work, split shifts, and variable schedules that better matched demand patterns.
Real-Time Management System: They implemented dashboards and alert systems that provided supervisors with visibility across all locations, highlighting exceptions requiring attention rather than overwhelming them with routine data. This system enabled more proactive management of service levels and performance issues.
The results of this implementation were substantial:
- 15% reduction in overall staffing costs through more efficient resource allocation
- 20% improvement in service level consistency across locations and time periods
- 12% increase in first-contact resolution due to better matching of interactions to agent skills
- 18% reduction in attrition through more employee-friendly scheduling and workload distribution
Perhaps most significantly, the data-driven approach revealed that their Colombia operation was particularly effective for certain high-value customer segments, leading to a strategic expansion of this location rather than the cost-driven offshoring they had originally planned.
The Future of Data-Driven Workforce Management
As data science, artificial intelligence, and computing capabilities continue to advance, several emerging trends will shape the future of workforce management in multinational contact centers:
Hyper-Personalized Agent Experience
Future systems will move beyond basic scheduling preferences to create truly personalized work experiences:
Cognitive and Emotional State Optimization: Routing and scheduling based not just on skills but on each agent’s current cognitive and emotional state, optimizing both performance and wellbeing.
Personalized Development Journeys: Automated identification of optimal learning paths for each agent based on their performance patterns, learning style, and career aspirations.
Work-Life Integration: Scheduling algorithms that consider each agent’s personal circumstances and preferences, creating truly individualized work arrangements that maximize both satisfaction and performance.
These capabilities will be particularly valuable for managing multinational workforces with diverse cultural expectations and personal priorities.
Predictive Experience Management
Beyond reactive staffing, future systems will anticipate and prevent customer experience issues:
Proactive Volume Intervention: Identification of potential volume spikes with sufficient lead time to implement preventive measures, such as proactive customer communications or temporary self-service enhancements.
Experience Risk Prediction: Real-time identification of interactions at risk of poor outcomes, with automated interventions to improve the customer experience before problems occur.
Optimal Experience Routing: Assignment of interactions based not just on efficiency but on predicted customer satisfaction and loyalty outcomes, optimizing for long-term value rather than short-term metrics.
These capabilities will enable contact centers to shift from managing service levels to actively shaping customer experiences across their multinational operations.
Augmented Workforce Intelligence
Advanced AI will increasingly complement human capabilities in workforce management:
AI-Assisted Decision Making: Intelligent systems that provide supervisors and managers with specific recommendations for addressing complex operational challenges, along with the reasoning behind those recommendations.
Autonomous Optimization: Self-adjusting systems that can make routine staffing and routing adjustments without human intervention, while escalating unusual situations for human judgment.
Scenario Planning Automation: AI-driven generation and evaluation of multiple operational scenarios, helping leaders make more informed strategic decisions about their global workforce configuration.
These capabilities will transform the role of contact center leaders from tactical problem-solvers to strategic experience architects, particularly in complex multinational environments.
Integrated Business Alignment
Future workforce management will be more tightly integrated with broader business objectives:
Revenue Optimization Modeling: Staffing and routing decisions that explicitly consider revenue potential and customer lifetime value, not just service level and efficiency metrics.
Brand Alignment Calibration: Workforce optimization that accounts for brand positioning and customer experience promises, ensuring that staffing decisions support the organization’s market differentiation.
Strategic Capability Development: Workforce planning that builds specific capabilities needed for future business strategies, rather than simply meeting current operational requirements.
For multinational operations, this integration will enable more strategic decisions about which functions to locate in which countries based on a sophisticated understanding of the unique value each location can provide.
The Strategic Imperative of Data-Driven Workforce Management
For organizations operating multinational contact centers, the transition to data-driven workforce management represents not merely an operational improvement but a strategic imperative. In an environment where customer experience increasingly determines competitive success, the ability to deploy the right people with the right skills at the right times across a global footprint has become a critical differentiator.
The organizations that excel in this capability gain multiple advantages:
Operational Efficiency: More precise alignment of staffing with demand across all locations, channels, and time periods, reducing both overstaffing and understaffing scenarios.
Customer Experience Quality: Consistent service levels and more effective interaction handling, leading to higher satisfaction, loyalty, and advocacy.
Employee Engagement: More stable workloads, fairer schedules, and better matching of tasks to skills, resulting in higher satisfaction and lower attrition.
Strategic Flexibility: Greater ability to adapt to changing business needs, customer expectations, and market conditions across diverse geographic locations.
For BPO operations in nearshore locations like Colombia, data-driven workforce management enables organizations to fully leverage the unique advantages these locations offer—from cultural affinity and language skills to time zone alignment and cost efficiency—while mitigating potential challenges through precise planning and real-time optimization.
The journey to truly data-driven workforce management requires significant investment in technology, analytics capabilities, and change management. However, the returns on this investment—in both financial performance and customer experience quality—make it essential for any organization seeking to compete effectively in today’s experience-driven marketplace.
By embracing the power of data to optimize their multinational contact center operations, forward-thinking organizations can transform what was once viewed as a cost center into a strategic asset that delivers sustainable competitive advantage.
PITON-Global connects you with industry-leading outsourcing providers to enhance customer experience, lower costs, and drive business success.
Digital Marketing Champion | Strategic Content Architect | Seasoned Digital PR Executive
Jedemae Lazo is a powerhouse in the digital marketing arena—an elite strategist and masterful communicator known for her ability to blend data-driven insight with narrative excellence. As a seasoned digital PR executive and highly skilled writer, she possesses a rare talent for translating complex, technical concepts into persuasive, thought-provoking content that resonates with C-suite decision-makers and everyday audiences alike.
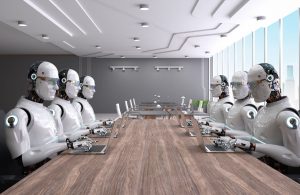
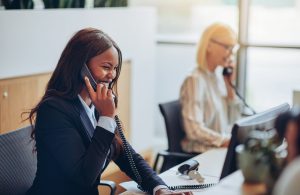
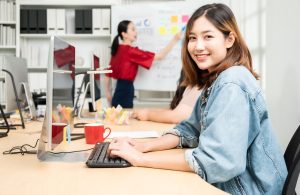